Data Science
Definition:
Data Science combines math and statistics, programming, AI, and machine learning with specific subject matter expertise to uncover insights hidden in data, especially large data sets.
Check out What is Data Science Education? SBOE Guide for more.
Practical Applications
“Data is the new oil,” and the primary fuel of many emerging technologies from artificial intelligence to blockchain. Yet while the technology landscape is highly dynamic, we can confidently predict that data will be a critical foundation for human exchange and economic value for the next several decades, if not permanently. Whether making daily decisions in personal finance or health, or helping create the next cutting-edge breakthroughs in academic research, every single person in our country would benefit from a strong foundation in the basics of data across contexts.
Three Examples of how a professional or person might use Data Science:
A farmer may need to combine data from multiple sources (soil-quality sensors, seasonal weather patterns, tractor equipment) to accurately predict crop yields and make critical decisions about go-to-market strategies. New programs for agricultural data science are driving both top-down changes in agriculture and quickly transforming the daily experience of U.S. farm owners.
A line-supervisor in an advanced manufacturing plant may need to work with an AI algorithm to ensure proper adjustments are made to packaging and shipping processes, and quickly interpret quality-control data or adjust faulty inputs to prevent multi-million dollar errors.
A nurse may need to query large patient databases for specific records to trace prior pre-existing conditions, blood type, or other background information, whether to improve a diagnosis or quickly triage an emergency room operation. More broadly, many healthcare institutions are making rapid advances in predictive care to reduce costs and improve health outcomes for patients at-scale.
Are there SPECIFIC standards for Data Science?
No, these are under development.
Are there places where Data Science appears in other standards?
A recent 2022 National Academies convening on the “Foundation of Data Science for K-12 Students” examined existing K-12 learning frameworks, and found many partial building blocks throughout existing K-12 subjects, including computer science. However, these existing frameworks have yet to be unified or modernized, and are often given little real time in the classroom due to competing content expectations.
The 2020 GAISE II (Guidelines in Assessment & Instruction for Statistics Education) from the American Statistical Association provides a critical building block for K-12 data science experiences, forwarding clear grade-span expectations for statistics education and data literacy. However, these guidelines have yet to be updated for modern technology changes, nor handle concepts related to AI literacy, data wrangling, or other modern techniques connected to data-use in industry or other applied contexts. They have also yet to be meaningfully adopted in math expectations.
The 2016 K-12 Computer Science Framework built a foundational thread on “Data & Analysis,” which provides a baseline for CS educators to consider when building foundational computing learning experiences. The framework includes four sub-concepts outlining Data and Analysis from a Computer Science lens: 1) Data Collection 2) Data Storage 3) Visualization and Transformation and 4) Inference and Models. The standards in the K-12 Computer Science Framework emphasize the management, treatment, and computational efficiency for analyzing data, and equip students to think about accuracy of computer models as a function of the quality and quantity of data – especially in the context of prediction and automation of data analysis (i.e. machine-learning). However, statistical inference, treatment of uncertainty, and other mathematics foundations are less emphasized in content expectations for CS. The Framework explicitly references Data Science: “Data science is one example where computer science serves many fields. Computer science and science use data to make inferences, theories, or predictions based upon the data collected from users or simulations. In early grades, students learn about the use of data to make simple predictions. As they progress, students learn how models and simulations can be used to examine theories and understand systems and how predictions and inferences are affected by more complex and larger data sets.”
The 2013 College, Career & Civic Life (C3) Framework for Social Studies Standards mentions “data” over 53 times throughout the standards. “Data” appears in a diverse set of contexts, including as one component of historical evidence (along with primary sources, documents, text, images, and other artifacts), as domain-relevant information for geography or economics, and in the context of geospatial technology and geographic information systems (GIS) (National Council for Social Studies, 2013). In formal standards, data is expected to be integrated into specific social studies courses, including Economics, Geography, Psychology, and Sociology.
While written over a decade ago, the 2011 Next Generation Science Standards (NGSS) recommends the inclusion of data science foundations in explicit terms: students are expected to “use digital tools (e.g., computers) to analyze very large data sets for patterns and trends”) as early as Grades 6-8 in Practice 5. Content expectations for data analysis basics appear in cross-cutting Practices, including Practice 4 (“Analyzing and interpreting data”) and Practice 5 (“Using Mathematics and Computational Thinking”) (ibid), both in Appendix F – Science and Engineering Practices. However, the degree to which these expectations have been implemented or emphasized in state assessments needs further exploration.
What are good starting resources for a teacher or administrator?
Teachers or administrators should review the Data Science 4 Everyone Resource Hub, a community-driven to collate all known curriculum resources, classroom software tools, lesson plans, professional development opportunities in K-12 data-based learning. Funding opportunities can be found on the DS4E Opportunities page. Both pages are updated regularly.
For Statistics / Mathematics educators specifically, DS4E recommends looking at resources available from the American Statistical Association.
Are there any media or stories of Data Science in education?
There has been a lot of schools and states integrating data science into K-12 education. Check out these stories:
-
EdWeek article on state-level work in Virginia
-
EdWeek article on state-level work in Utah
-
EdWeek article on rigor of teaching with data
-
Hechinger op-ed on data science
-
Hechinger article on state-level work in North Carolina
-
The Hill op-ed on data illiteracy
See https://www.datascience4everyone.org/news for the latest news and updates!
How do ethics and social impacts intersect Data Science?
Teaching ethics in data science is critical–from data privacy to misinformation to algorithmic bias–and is a fundamental component of K-12 data science education goals across programs. While field-wide learning expectations are underway for K-12 Data Science, work facilitated by the Academic Data Science Alliance (ADSA)’s Project Ethos is driving greater emphasis of ethics, privacy, and responsible use of data in the academic field of data science, including for undergraduate data science education. K-12 continues to work with higher-education partners to ensure age-appropriate translation and expansion of this work, so that students know how to navigate the increasingly complex landscape of technology ethics.
What keywords could be searched to find out more about Data Science?
Data Science
Data Literacy
K-12 Data Science
How can we build capacity to add this field to our instruction?
Some CS or Math curricula have lessons or resources for teaching about data, but may not cover the full scope of data science. Check with your favorite curriculum or platform, such as Bootstrap, Introduction to Data Science, brainPOP, Learning.com, or others, to see what is available and use the member curriculum page at CSforALL.org or the resources page at Data Science 4 Everyone to find others.
Financial Support
Data science can be aligned with many existing subject areas like math, science, or social studies. Look for funding for new and novel approaches to teaching existing subjects, and inspiration from project examples from the internet. Also check-out the DS4E’s Guide on Leveraging Federal Funding for pilot programs, teacher training, and more.
Pathway planning and Support:
-
Contact the DS4E team here for assistance with pilot and program design.
-
Already started a program at your school or district? Tell us about it here.
-
Sign up for the CSforALL SCRIPT Program for peer workshops in standards-complete CS education.
-
Check with your local CSTA chapter or with your state department of education for upcoming professional learning and pathway planning opportunities.
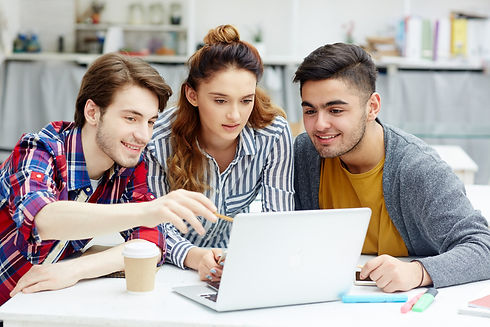
How Its Connected
Data is at the heart of modern computing, but without algorithms and programming we would not be able to analyze the large data sets that give AI its power. GPT and other large language models are possible because of techniques in data science which rely heavily on math and statistics.